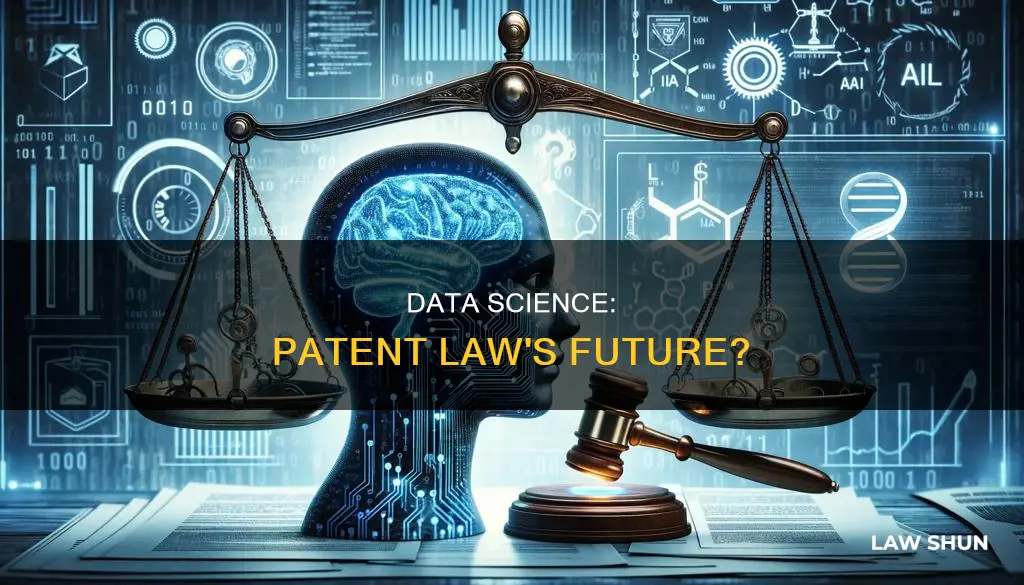
Data science and patent law are two very different fields, but they do intersect in some interesting ways. Data science methods can be used to analyze complex business environments, especially in innovation-driven industries, and patents are a primary data source for technological information. On the other hand, patent law is a well-established process to protect innovative products and processes, and it involves a lot of paperwork and legal knowledge. This article will explore the relationship between data science and patent law, including the benefits and challenges of each field, and how they can work together to create value for organizations.
Characteristics | Values |
---|---|
Data science methods and patents | Data science methods can be used to analyze patents and provide great benefits to companies. |
Patent law and data science | Patent law is a difficult field to get into and has more job security than data science. |
Data science career | Data science is a hard field to break into due to lots of competition. |
Patent law career | Patent law has a higher starting pay than data science. |
Patenting a data science method | Patenting a data science method may not always be a good idea as it may be difficult to detect infringement. |
ML and patents | A new invention that uses ML in a different way may be patentable. |
What You'll Learn
Data science patent applications
A patent provides the right to exclude others from using your inventions. However, to execute that right, you first need to know when someone is using your inventions. This is not always straightforward or even possible in data analytics and related fields. For example, if someone takes your method, implements it using some language, compiles it and commercializes the executable, then there is no way to find out if your patent is being infringed upon. Moreover, you have to disclose the method in order to claim the right on the same, meaning your patent application becomes a white paper for your competitors.
There are many ways to find out if your invention is in use. For example, your method can enable the processing of two completely different data sources that were not possible before, or your new method can reduce a few steps in the whole workflow of data processing. Once you find such points, make claims of your patent application based on such points only. If you cannot find such points, which is very likely for the data analytics method, then keep quiet and make it a trade secret.
ML patent applications must be drafted with at least one well-described practical example, such as faster search, better search, linguistics analysis, or better weather forecasting. As a general rule, the more you can describe in the patent application, the better it will be. Another way to help with patent-eligibility is to describe the technical details of the implementations, which is not too difficult given the technical nature of ML. For example, describing how to vectorize data, combine multiple fields, or perform dimension reduction can assist with patent-eligibility.
Mother-in-Law Visa: Can Citizens Apply?
You may want to see also
The value of data science patents
Data science patents can be extremely valuable, as demonstrated by Larry Page's "PageRank" patent application (US6285999 B1), which is considered one of the most profitable data science patents to date. However, the process of obtaining a patent can be complex and costly, and there are concerns about the effectiveness of patents in protecting data science inventions.
One of the main challenges in patenting data science methods is detecting infringement. It can be difficult to determine whether someone has implemented and commercialized your method, especially in the field of data analytics. Additionally, the patent application process requires disclosing the method, which can provide competitors with valuable information. This, combined with the significant time and monetary investment, can make patenting a risky proposition for independent inventors.
Despite these challenges, data science patents can provide significant benefits. They offer the right to exclude others from using your inventions, which can be crucial in maintaining a competitive edge. Additionally, patents can serve as a form of recognition and validation for data science accomplishments, which are often overlooked or undervalued.
Furthermore, the application of data science techniques to patent data can provide valuable insights for companies. Visualizing different aspects of patent data can help identify key players and technology areas, understand the development stage of a research field, and make more informed investment decisions. Data science methods can also facilitate the analysis of complex business environments, enabling companies to gain insights into emerging topics, research efforts, and the potential of specific technology fields.
In conclusion, while there are challenges associated with data science patents, they can provide significant value. Patents offer protection for inventions, recognition for data scientists, and valuable insights for businesses. However, inventors should carefully consider the potential risks and costs before pursuing a patent, especially in the case of independent inventors with limited resources.
Congress' Self-Exemption: Legal or Immoral?
You may want to see also
Data science vs patent law career paths
Data science and patent law are two distinct career paths that offer a range of opportunities and challenges. Both fields are highly specialised and require a unique set of skills and qualifications. When choosing between the two, it is essential to consider the work environment, job prospects, income, job security, and work-life balance offered by each career path.
Data science is a broad term that encompasses various roles, including data analysts, data engineers, machine learning engineers, and data scientists. Data scientists, for example, are responsible for collecting, analysing, and interpreting large sets of data to derive meaningful insights and drive decision-making within an organisation. This field requires strong technical skills in areas such as programming, statistics, and machine learning, as well as soft skills such as problem-solving and communication. Breaking into data science can be challenging due to the competitive nature of the field and the constant evolution of technology. However, those with valuable skills, such as machine learning, will always be in demand. Additionally, data science often involves working in teams and offers a better work-life balance compared to patent law.
On the other hand, patent law is a legal field that deals with the protection of intellectual property rights for inventions. Patent lawyers, also known as patent attorneys, assist clients with patent applications, prosecution, and maintenance. They work closely with scientists, engineers, and innovators to understand their inventions and advocate for their patentability. Patent law requires a strong understanding of scientific and technical concepts, excellent communication and writing skills, and the ability to work independently and manage projects. Becoming a patent lawyer typically involves completing a law degree, passing the bar exam, and gaining practical experience through internships or training at a law firm. Patent law offers higher starting pay than data science, but it also comes with longer working hours and a more demanding work environment.
In terms of job prospects, data science jobs may be more susceptible to industry fluctuations, as evident in the current challenges faced by the tech industry. However, the field of data science is constantly evolving, and skilled professionals are always in demand. Patent law, on the other hand, offers long-term job security, especially in the US, where there will always be a need for lawyers.
When it comes to income, patent law typically offers higher earning potential, especially at law firms, where billing plays a significant role in determining earnings. Data scientists can also earn competitive salaries, with experienced professionals earning in the range of 200k. However, the income potential in data science may vary based on the specific role, organisation, and demand for specialised skills.
In summary, both data science and patent law offer rewarding career paths with unique advantages and challenges. Data science provides a broader range of opportunities, a better work-life balance, and strong job security for those with in-demand skills. Patent law, on the other hand, offers higher earning potential, long-term job security in the legal field, and the opportunity to work with innovative scientists and engineers across various fields. The choice between the two ultimately depends on individual interests, skills, and career aspirations.
Gold Dot Ammunition: Available to Both Civilians and Law Enforcement
You may want to see also
Data science methods for patent analysis
Patent analysis is a comprehensive process that involves examining and evaluating patents to gain insights into various aspects of innovation, technology trends, competitive landscapes, and intellectual property. It is a multidisciplinary field that combines technology assessment, business strategy, legal expertise, and data analysis to extract valuable insights from patent documents and shape innovation strategies.
Data science methods have been increasingly used in patent analysis since around 2020. These methods include machine learning, semantic technologies, and artificial intelligence. Machine learning, for example, can be used for keyword searching, classification searching, and citation analysis, and the semi-automated production of visualizations, dashboards, and reports. The use of machine learning in patent analysis has improved efficiency and scalability.
One challenge in patenting data science methods is detecting infringement. For instance, if someone takes your method, implements it using a different language, compiles it, and commercializes it, there may be no way to find out. Additionally, the patent application process can be costly and time-consuming, and there is a risk that the method will become public knowledge without providing any tangible benefits.
However, there are successful examples of data science patent applications, such as the "PageRank" patent application (US6285999 B1) from inventor Larry Page, which has been highly profitable. When considering patenting a data science method, it is important to carefully evaluate the potential benefits and drawbacks and to ensure that the method provides a new interface for interaction with people and other entities, like sources of data.
Writing Laws: Citizen Power to Draft Bills
You may want to see also
Patent data analytics tools
One such tool is LexisNexis Intellectual Property Solutions, which provides access to extensive patent databases through its search tool, LexisNexis TotalPatent One®. This tool offers full text, bibliographic, and abstract data from numerous authorities, ensuring users have access to comprehensive and up-to-date information. LexisNexis also offers PatentSight®, which provides unique metrics and analytical capabilities to help decision-makers navigate their patent portfolios and identify valuable patents for licensing.
Another notable patent data analytics tool is Solve Intelligence, which ranks highly among AI patent analysis tools. Solve Intelligence covers every stage of the patenting process, including patent drafting, prosecution, and invention disclosure submission. It offers features such as figure generation, figure drafting, and editing, as well as the ability to input chemical structures and tabular data, catering to a wide range of technological fields.
InnovationQ Plus is another IP intelligence software that delivers competitive insights and data analytics. This tool enables engineers, researchers, and IP professionals to make informed decisions about intellectual property. Lens is a free search and analysis tool that helps users discover, analyze, and map global innovation knowledge. It offers advanced search functions, filtering, and sorting options, enhancing the efficiency of the patent search process.
PatentsView is a unique open data platform that provides data dissemination and value-added analyses, fostering a deeper understanding of the IP system and driving new insights into invention and innovation. Additionally, the WIPO IP statistics data center is an online service providing access to worldwide IP activity data, allowing users to select relevant indicators and download the latest and historical data.
These patent data analytics tools empower users in the patent law field to make strategic decisions, identify trends, manage portfolios, and stay informed about the dynamic world of intellectual property.
Congress's Power: Can They Repeal State Law?
You may want to see also
Frequently asked questions
Data science methods can be used to analyse patents, which can create great benefits for companies. Patents are a primary data source for technological information that is well-suited to be analysed by data science methods.
Data science methods can be used to break down complex industry environments, enabling organisations to be allocated to specific segments. For example, data science methods can be used to analyse which organisations dominate the battery market by dividing the market by battery components.
Patenting a data science method can be challenging because it may be difficult to detect any infringement. Additionally, the inventor must disclose the method in order to claim rights over it, essentially providing a white paper for competitors.
Data science offers a better work-life balance than patent law, as the latter involves billable hours which can be painful, especially when trying to make up hours due to illness. Patent law has a higher starting pay than data science, but it also involves significantly more work.