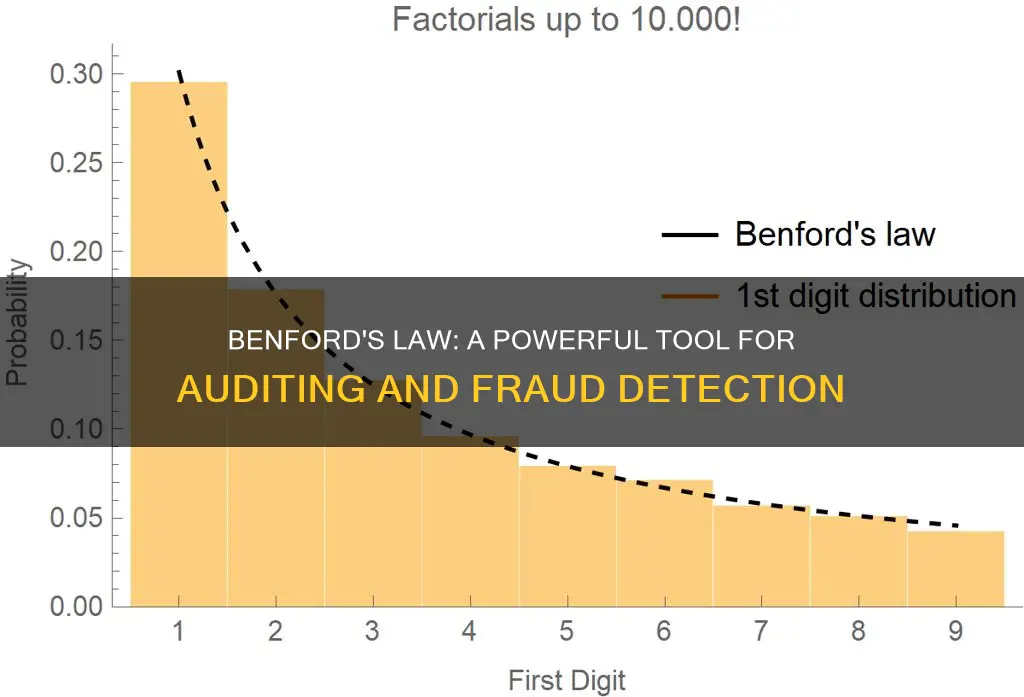
Benford's Law is a mathematical theory that can be used to detect fraud in accounting and auditing. It states that in a naturally occurring set of numbers, smaller digits appear more frequently as the leading digit. For example, the number 1 appears as the first digit about 30% of the time, while 9 appears less than 5% of the time. This law can be applied to large data sets that grow exponentially, such as accounting transactions, to identify potential fraud or anomalies. Auditors can use spreadsheet programs to analyse the distribution of digits and calculate the frequency of each digit from 1 to 9. While Benford's Law is a useful tool, it has limitations and may not be suitable for all types of data. It is important to understand the constraints and applicability of Benford's Law before applying it in auditing to ensure reliable results.
Characteristics | Values |
---|---|
Use | Detecting fraud, testing journal entries for unusual numeric patterns |
Leading digits | Numbers beginning with smaller digits occur more frequently |
Example | Numbers beginning with 1 occur about 30% of the time, numbers beginning with 2 occur about 18% of the time, and so on, down to numbers beginning with 9, which occur less than 5% of the time |
Applicability | Data sets that go across multiple orders of magnitude (e.g. populations of towns or cities, income distributions) |
Inapplicability | Data sets in which digits are predisposed to begin with a limited set of digits, e.g. human heights, weights and IQ scores |
Data set size | Large data sets are preferable, with a minimum of 5,000 records recommended |
Data type | Data must be numeric, randomly generated, large, and represent magnitudes of events |
Data examples | General ledgers, income statements, inventory listings, network traffic |
What You'll Learn
Detecting fraud
Benford's Law is a powerful tool for detecting fraud in accounting and financial data. It is based on the observation that in genuine data sets of numbers, the leading digit is likely to be small. This means that in a legitimate data set, the numeral "1" will be the leading digit about 30% of the time, "2" will be the leading digit around 18% of the time, and so on, with the frequency decreasing as the digits get larger. This results in a downward-sloping curve, known as the Benford curve, which can be used as a baseline to identify anomalies in financial data that may indicate fraud.
To apply Benford's Law in auditing, auditors typically use spreadsheet programs to analyse the distribution of digits in a random set of numbers. By calculating the frequency of each digit from 1 to 9, auditors can identify any significant deviations from the patterns predicted by Benford's Law. For example, if 20% of the numbers in a data set begin with "9" and only 10% begin with "1", this may indicate financial misstatement. However, it is important to note that such deviations do not prove wrongdoing, as there may be innocent explanations such as human error.
Benford's Law is particularly useful for detecting fraud in the following areas:
- Accounts payable or receivable
- General ledgers
- Credit card transactions
- Disbursements
- Inventory prices
- Journal entries
- Loan data
- Purchase orders
- Stock prices
- Tax assessments
- Insurance claims
When applying Benford's Law, it is important to consider certain constraints. The theory works best with large data sets that are randomly generated and span multiple orders of magnitude. It may not be suitable for small data sets, data with pre-arranged or artificial limits, or data generated by formulas or human choices. Additionally, Benford's Law sometimes generates false positive and negative results, so auditors should be cautious and consider alternative audit procedures to assess the validity of questionable data.
Shipping Laws: Home Brew and Wine, What's the Difference?
You may want to see also
Testing journal entries
Step 1: Understanding Benford's Law
Benford's Law is a mathematical theory that describes the distribution of leading digits in datasets. According to this law, in random data, numbers beginning with smaller digits occur more frequently. For example, numbers starting with the digit "1" occur about 30% of the time, while numbers starting with "9" occur less than 5% of the time. This law can be applied to analyse the first digit, second digit, first two digits, and other combinations.
Step 2: Data Preparation
When testing journal entries, auditors should obtain the client's general ledger and ensure it agrees with the year-end trial balance. Identify the total number of line items in the general ledger, as this will determine the expected count distribution. Collapse debits and credits into one column, keeping both sides positive. Ensure the dataset is in a format that can be analysed, such as a spreadsheet.
Step 3: Calculating Expected Distribution
To calculate the expected distribution of leading digits, auditors can use the formula =(LOG(1+(1/A))/LOG(10)), where "A" refers to the cell containing the value in the dataset. This formula will provide the expected percentage for each of the possible leading digits. The sum of these percentages should equal 100%.
Step 4: Identifying Leading Digits
Use spreadsheet functions to identify the leading digit on every line of the dataset. Adjustments may be needed for entries less than a dollar by ignoring leading zeros and manually keying in the first digit. For example, $0.03 would have "3" as the first digit.
Step 5: Calculating Deviations
Calculate how often each digit appears as the leading digit and identify the count and percentage variances from the expected distribution. Determine if any deviations are statistically significant using Z-scores or other statistical methods. A large difference between the expected and observed percentages may indicate potential fraud or anomalies.
Step 6: Investigating Anomalies
If significant deviations are identified, auditors should investigate further. Consider red flags such as weekend entries, off-hours entries, or debits to revenue accounts. Select specific entries with leading digits that have significant deviations for further analysis. Use professional judgement and consider the context of the transactions.
Step 7: Interpreting Results
While deviations from Benford's Law may indicate potential fraud, they can also be due to legitimate business practices or random fluctuations. If anomalies are identified, auditors should perform additional review procedures to determine the underlying causes. Consider alternative audit procedures, such as tracing transactions to supporting documentation or comparing to prior years' data.
Step 8: Considerations and Limitations
Keep in mind that Benford's Law may not be suitable for all types of journal entries. It is most effective for manual entries used for adjustments, accruals, and rare activities. Additionally, ensure the dataset is large enough, as Benford's Law is most reliable with datasets of at least 5,000 records. Be cautious of confounding variables, such as small data sets, assigned numbers, and artificial limits, which can affect the accuracy of the analysis.
English Law in the US: Who Rules?
You may want to see also
Identifying general ledger irregularities
Benford's Law can be used to identify irregularities in general ledgers. It is a mathematical theory of leading digits, which states that in data sets, the leading digit(s) are distributed in a specific, nonuniform way. For example, the number 1 appears as the first digit about 30% of the time, whereas 9 is the first digit less than 5% of the time.
Benford's Law can be applied to identify general ledger irregularities, both those that indicate fraud and those that do not. Deviations from Benford's Law could indicate the presence of large counts of fictitious journal entries below the auditor's testing threshold or unusually high duplications of same-dollar transactions. These irregularities may be due to processing inefficiencies or high duplications of per diem travel allowances, and are not necessarily fraudulent.
To identify these irregularities, auditors can use data analytics techniques such as the Runs test to detect ridges and valleys in the results, and also identify actionable spikes. Benford's Law-based testing can direct auditors to large counts of journal entries that are below their testing threshold, which could indicate fraud.
Benford's Law is most effective when applied to large data sets, with a minimum of 5,000 records. It is recommended that the data set be 1,000 records or more, as using fewer than 1,000 can lead to too many false positives. The data should be randomly generated, without any real or artificial restrictions on the occurrence of digits.
Benford's Law is not suitable for all types of journal entries. It should not be used for data sets with a maximum or minimum number, such as human heights or weights, or data generated by formulas. It is also not suitable for small data sets of 500 or fewer transactions.
Police and HIPAA: Understanding Legal Boundaries and Applicability
You may want to see also
Analysing credit card transactions
Benford's Law can be used to analyse credit card transactions for unusual patterns that may indicate fraud. The law states that in sets of random data, numbers beginning with smaller digits occur more frequently. For example, numbers beginning with 1 occur about 30% of the time, while numbers beginning with 9 occur less than 5% of the time.
When applying Benford's Law to credit card transactions, auditors typically use a spreadsheet program to examine the distribution of digits in random sets of numbers. They calculate the frequency with which the digits 1 through 9 occur and convert the spreadsheet into a chart that highlights any significant deviations from the predicted patterns. For example, if 20% of the numbers in a dataset begin with 9 and only 10% begin with 1, this may indicate financial misstatement.
However, it is important to note that Benford's Law does not always prove wrongdoing. Suspicious patterns can often be explained by innocent factors such as duplicate entries and other human errors. Additionally, Benford's Law sometimes generates false positive and negative results, especially with small datasets.
To apply Benford's Law effectively in the analysis of credit card transactions, auditors should ensure that the dataset is large enough to be statistically relevant. The data should also be naturally occurring, as the law does not work for non-random numbers like invoice numbers. It is also important to consider any artificial limits on transactions, such as maximum or minimum thresholds, which may affect the distribution of digits.
By using Benford's Law to analyse credit card transactions, auditors can identify unusual numeric patterns that may indicate fraud or errors. This technique provides a simple and cost-effective tool to enhance financial reporting and detect potential issues.
Constitutional Law: Student Rights and Their Protection
You may want to see also
Testing controls
When applying Benford’s Law in auditing, it is important to consider the specific controls that may impact the effectiveness of the analysis. Controls are the policies and procedures that a company has in place to ensure the accuracy and integrity of its financial data. If these controls are not properly designed or effective, it could impact the reliability of the financial data and the results of the Benford’s Law analysis.
One key control to consider is the process for recording and approving transactions. For example, if a company requires that all transactions above a certain threshold be approved by a manager, this control should be tested to ensure it is operating effectively. This could involve reviewing a sample of transactions above the threshold to ensure that proper approval was obtained. If it is found that transactions are routinely approved without proper review, it could indicate a breakdown in controls that could impact the reliability of the financial data.
Another important control to test is the process for reconciling accounts and identifying and correcting errors. Auditors should review the process for reconciling financial data and identifying and addressing any discrepancies. If it is found that discrepancies are routinely ignored or not properly investigated, it could indicate a control breakdown that could impact the integrity of the financial data.
Additionally, the control environment surrounding the financial reporting process should be evaluated. This includes considering the competence and training of staff, the tone set by management, and the overall culture of the organization. For example, if staff lack adequate training on accounting procedures, it could lead to errors in financial data that impact the results of the Benford’s Law analysis. Similarly, if management places undue pressure on meeting financial targets, it could create an environment where employees feel compelled to manipulate financial data.
Overall, when applying Benford’s Law in auditing, it is crucial to assess the effectiveness of the controls in place. By testing these controls, auditors can help ensure the reliability of the financial data and enhance the effectiveness of the Benford’s Law analysis as a tool to identify potential anomalies.
HIPAA Laws: Who's Watching the Board of Examiners?
You may want to see also
Frequently asked questions
Benford's Law, named after physicist Frank Benford, describes the distribution of leading digits in data sets. It states that in a naturally occurring set of numbers, smaller digits appear more frequently as the leading digit. For example, the number 1 appears as the leading digit about 30% of the time, while 9 appears less than 5% of the time.
Benford's Law can be used to detect anomalies and potential fraud in financial data. By comparing the distribution of leading digits in financial data to the expected distribution according to Benford's Law, auditors can identify suspicious patterns that may indicate fraudulent activity or accounting errors.
Benford's Law has some limitations. It is most effective for large data sets with naturally occurring numbers. It may not be suitable for small data sets, assigned or sequential numbers, or data with artificial limits or restrictions.
Auditors can use Benford's Law to analyze general ledger entries, accounts payable and receivable, and other financial transactions. For example, they can compare the frequency of leading digits in disbursements to the expected probabilities to detect potential fraud or errors.
Benford's Law assumes that naturally occurring data will follow a specific distribution of leading digits. If someone manipulates financial data, they are likely to create randomly generated numbers that deviate from this distribution, making it a powerful tool for fraud detection.