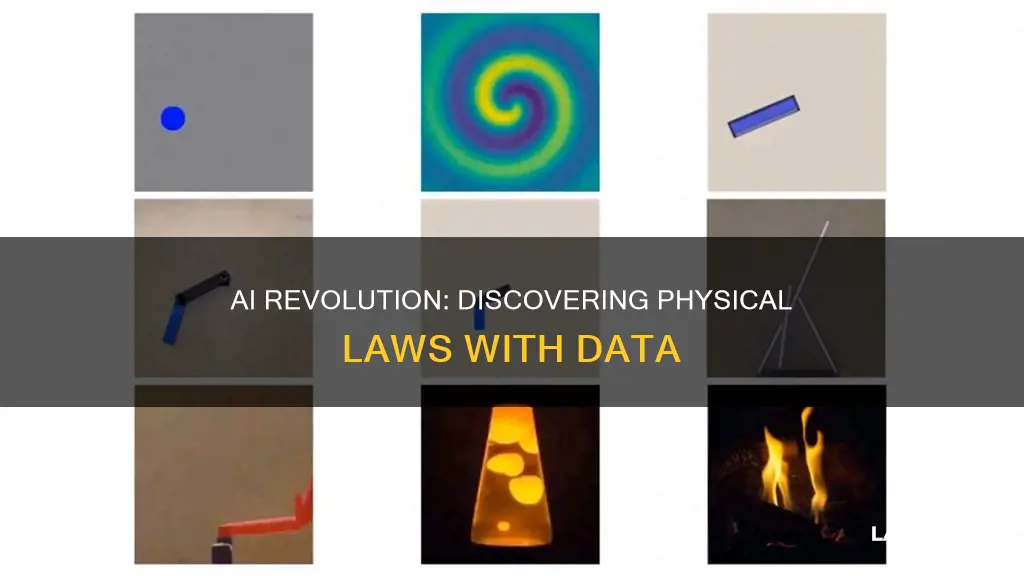
Artificial intelligence (AI) and machine learning are transforming physics research, with scientists using these technologies to discover hidden physical laws and equations of motion in various data. AI can identify patterns and correlations in vast datasets, such as those from particle accelerators, that might indicate the presence of new particles or forces. This has led to the development of methods to discover novel equations of motion in observational data, which can then be used to create models and simulations that are faithful to the laws of physics. This technology could enable the discovery of hidden equations of motion behind phenomena that were previously considered unexplainable, such as ecosystem changes. AI may not replace human physicists, but it can augment their ability to analyze data and spot anomalies, guiding the way toward new theories and laws.
Characteristics | Values |
---|---|
AI's role in physics research | AI is transforming physics research by merging the rigour of scientific inquiry with the speed and adaptability of machine learning algorithms. |
AI as a physicist | With access to vast datasets from particle accelerators, astronomical observatories, and climate models, an AI physicist can identify patterns that humans might overlook. |
AI as a tool | AI can serve as a powerful assistant, sifting through data and highlighting promising leads for physicists to investigate further. |
AI's ability to discover physical laws | AI can discover hidden physical laws in various data. |
AI's ability to identify patterns | Neural networks could recognise patterns in gravitational wave data or particle collision results and infer the presence of new physical phenomena. |
AI's ability to analyse data | AI excels at parsing vast datasets, identifying patterns, and finding correlations that might signal the presence of new particles or forces. |
AI's ability to speed up discoveries | AI-enhanced simulations can provide insights into systems that are impossible to study directly, vastly accelerating the pace of discovery. |
AI's ability to simplify calculations | AI can learn from the data of specific phenomena and construct small-scale models, simplifying and speeding up calculations that are faithful to the laws of physics. |
AI's ability to improve models | AI can produce highly reliable models from observational data of phenomena. |
What You'll Learn
AI's ability to recognise patterns in data
At its core, pattern recognition in AI involves teaching computers to recognise patterns in data so that they can make intelligent decisions. This is achieved through the use of training data, which includes examples relevant to the task the AI is learning to perform. The AI system then extracts features from the data, such as distinctive attributes or aspects, and uses these to identify and classify patterns.
One example of AI's pattern recognition capabilities is in the field of physics, where AI models are being used to discover hidden physical laws and equations of motion in observational data. For instance, researchers have developed a neural network deep learning technique to extract hidden turbulent motion information from observations of the Sun. This technology could enable the discovery of hidden equations of motion behind phenomena that were previously considered unexplainable, such as ecosystem changes.
In addition to physics, AI's pattern recognition abilities have revolutionised medical diagnostics, improved security systems, and enhanced simulations in fields such as fluid dynamics, astrophysics, and materials science. For example, AI-enhanced simulations can provide insights into systems that are impossible to study directly, such as the behaviour of matter near a black hole or the conditions that led to the Big Bang.
While AI may not replace human physicists, it can augment their abilities to analyse data and spot anomalies, guiding the way towards new theories and laws. The combination of human intuition and machine intelligence creates a formidable partnership in the quest for scientific discovery.
Federal Regulations: Overriding State Law?
You may want to see also
The need for human-machine collaboration
The marriage of artificial intelligence and physics is a powerful one, with AI able to rapidly process and identify patterns in vast datasets, and physics providing the framework for discovery. AI is already transforming physics research, but it is not a replacement for human physicists. Instead, it is a tool that can augment human capabilities, speeding up the process of discovery and providing valuable insights.
AI has been successfully used to discover hidden equations of motion in observational data, creating models that are faithful to the laws of physics. This has been achieved without the need for experimental objects or real data, and it may be possible to use this technology to discover the hidden equations of motion behind phenomena that were previously considered unexplainable. For example, it may be possible to use physics-based knowledge and simulations to examine ecosystem sustainability.
However, there are challenges to overcome. Machine learning models often struggle with learning new physics and explaining predictions, and they are prone to errors when faced with complex secondary physical mechanisms, like unsteady fluid drag forces. The naive application of ML/AI will generally be insufficient to infer universal physical laws without further modification. It is also difficult to apply realistic data to these models, as it is often unclear what data format is best to use.
The human-machine collaboration is, therefore, necessary to address these challenges and push the boundaries of scientific inquiry. Humans can provide the intuition and theoretical framework, while machines can process vast amounts of data and identify patterns and correlations that might signal the presence of new particles or forces. This combination of human and machine intelligence has the potential to become a formidable partnership in the quest for discoveries, with AI casting a spell over the world of physics and expanding what we are capable of discovering.
Understanding Rate Laws: Concentration Over Concentration
You may want to see also
AI's role in simplifying complex calculations
AI is playing an increasingly significant role in simplifying complex calculations. AI-based models are being used to extract hidden equations of motion from observational data and create models that are faithful to the laws of physics. This technology can be applied to various fields, including physics, fluid dynamics, astrophysics, and materials science.
For example, researchers at Kobe University and Osaka University have developed AI technology that can extract hidden equations of motion from regular observational data. This enables the discovery of hidden equations of motion behind phenomena that were previously considered unexplainable, such as ecosystem sustainability.
AI is particularly useful in handling vast datasets, identifying patterns, and finding correlations that may indicate the presence of new particles or forces. For instance, particle accelerators like the Large Hadron Collider (LHC) produce petabytes of data annually, which cannot be manually analyzed. AI can efficiently parse through this data, making it a valuable tool for physicists.
Additionally, AI-enhanced simulations can provide insights into systems that are challenging or impossible to study directly. For example, AI models could potentially predict the behavior of exotic matter near black holes or simulate the conditions of the Big Bang. This capability allows researchers to experiment with more variables and explore a broader range of scenarios, accelerating the pace of discovery.
Furthermore, AI can assist in simplifying complex calculations by acting as a powerful assistant to physicists. It can sift through data, highlight promising leads, and guide researchers toward new theories and laws. This combination of human intuition and machine intelligence creates a formidable partnership in the quest for scientific discoveries.
Urban Legal Uniqueness: Can Cities Have Their Own Laws?
You may want to see also
The challenge of universal laws and discrepancies
One key challenge is the need to account for discrepancies between theoretical predictions and empirical observations. In the case of studying falling objects, for instance, factors like measurement noise and complex secondary physical mechanisms, such as unsteady fluid drag forces, can obscure the underlying laws of gravitation. This issue underscores the necessity of developing robust data-driven methods that can effectively disentangle these complexities and identify the governing physical principles.
To address these challenges, researchers have employed various techniques. For example, the sparse identification of non-linear dynamics (SINDy) method has been utilized to identify governing equations from real-world measurement data and simulated trajectories. Additionally, the concept of parsimony has been applied to machine learning systems, where "stochastic optimization" helps balance simplicity with accuracy, making it easier to extract meaningful physics from the data.
Furthermore, the application of geometry has been pivotal in developing AI capable of discovering hidden equations of motion within observational data. By illuminating the unknown geometric properties of phenomena, AI can extract these equations and create models and simulations that align with physical laws. This approach has the potential to revolutionize various fields, including weather forecasting, drug discovery, building analyses, and car design, by simplifying and accelerating calculations.
In conclusion, while AI and machine learning have expanded the boundaries of scientific knowledge, the challenge of universal laws and discrepancies remains a critical consideration. Addressing this challenge requires sophisticated methodologies that can navigate the complexities of real-world data and uncover the underlying physical principles. Through ongoing advancements and adaptations, AI continues to demonstrate its potential as a powerful tool for scientific discovery, augmenting human capabilities in the quest for new physical laws.
Counties' Power: Can They Override State Law?
You may want to see also
AI's potential to augment human intuition
AI has the potential to augment human intuition and transform physics research. AI can be used to automate the discovery of physics principles and governing equations from measurement data alone. AI can also identify underlying equations and patterns in data, which can lead to the discovery of new physical phenomena and laws of nature. For example, AI can be used to predict the behaviour of matter near a black hole or in the early universe, which could lead to the discovery of new laws of nature.
AI can also assist in analysing experimental data from particle accelerators like the Large Hadron Collider (LHC), which produces petabytes of data yearly that cannot be manually analysed. AI can identify patterns and correlations in this data that may signal the presence of new particles or forces. This can lead to more efficient and accurate simulations in fluid dynamics, astrophysics, and materials science, enabling researchers to experiment with more variables and explore more scenarios.
AI can also be used to develop models and simulations that are faithful to physical laws. For example, AI can extract equations of motion from observational data and create models that are consistent with the laws of physics. This can be applied to a wide range of fields, including weather forecasting, drug discovery, building analyses, and car design, simplifying and speeding up calculations.
However, there are challenges and limitations to using AI for discovering physical laws. One challenge is the need for a discrepancy model to account for the mismatch between theory and measurements. Naive application of AI techniques may not be sufficient to infer universal physical laws without further modification. Additionally, machine learning systems can struggle with learning new physics and explaining predictions, and may require large amounts of data and computational power.
Overall, AI has the potential to augment human intuition in physics research by automating data analysis, identifying patterns and correlations, and developing models and simulations consistent with physical laws. However, there are also challenges and limitations to its effectiveness that must be addressed.
Distracted Driving Laws: Enforceable or Not?
You may want to see also
Frequently asked questions
AI has the potential to discover new physical laws by recognizing patterns in data. AI can analyze large datasets and identify patterns that might indicate the presence of new particles or forces. However, it is challenging to posit a universal physical law from data without accounting for the mismatch between theory and measurements.
AI can discover new physical laws by using machine learning to interpret data and find underlying equations. This enables the creation of models and simulations that are faithful to physical laws.
AI can discover new physical laws much faster than traditional methods, which can take decades. AI can also handle large and complex datasets that would be difficult to analyze manually.