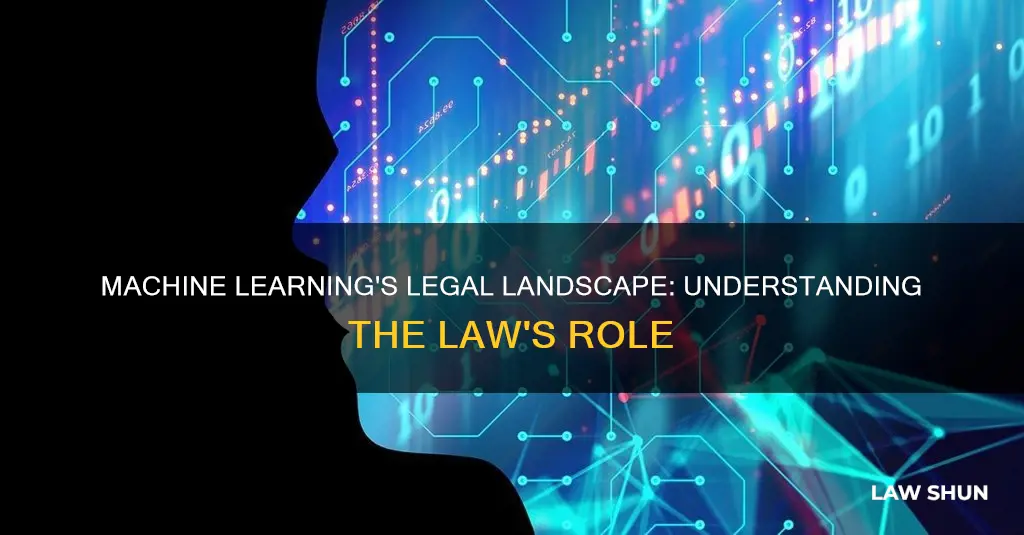
Machine learning is an increasingly important technology in the legal sector, with the potential to transform the work of lawyers and legal professionals. Machine learning is a subset of artificial intelligence (AI) that focuses on data and uses algorithms and statistics to identify patterns within large datasets. While it does not replace human expertise, it can augment it, allowing legal professionals to focus on providing the best counsel and advice to their clients.
In the legal sector, machine learning is being used for a range of tasks, including contract review, due diligence, and document management. It can also assist with more complex tasks such as litigation preparation and legal research. The use of machine learning in law is still evolving, and there are challenges to its implementation, including ethical considerations and the need for clear regulations. However, its potential to increase efficiency and improve access to justice makes it an important area of development.
Characteristics | Values |
---|---|
Purpose | To ensure machine learning systems are fair, transparent, and efficient |
Data | Huge datasets are required to train machine learning models effectively |
Human Input | Humans train machine learning systems on past experiences and industry-specific information |
Regulation | The European Union has passed a regulation giving citizens a "right to an explanation" for decisions made by machine learning systems |
Testing | Models should be rigorously evaluated on test datasets before deployment |
Accuracy | An accuracy level of 90% can bring huge benefits if the model is embedded in the right workflow |
Validation | A validation station is essential to confirm the predictions made by machine learning models |
Integration | Off-the-shelf solutions may have limited integration options, making it difficult to transfer results to another platform |
Deployment | Self-developed models require specialists to deploy, while off-the-shelf solutions may be available at a relatively low cost |
What You'll Learn
The right to an explanation for decisions made by machine learning systems
In the context of machine learning and the law, the "right to an explanation" refers to an individual's right to receive an explanation for decisions made by algorithms that significantly impact their lives. This right is especially pertinent when automated decisions have legal or financial implications. For instance, if an individual is denied a loan by a machine learning system, they have the right to request an explanation outlining the factors that contributed to the decision.
The "right to explanation" is a topic of ongoing debate, with arguments both for and against its implementation. Proponents of this right argue that it is a crucial foundation for an information society, promoting transparency and trust in automated decision-making systems. On the other hand, critics suggest that such a right may hinder innovation, favour human decisions over machine decisions, and lead to a focus on process rather than outcome.
The European Union's General Data Protection Regulation (GDPR), enacted in 2016 and taking effect in 2018, introduced provisions related to automated decision-making. While the regulation does not explicitly mention a "right to explanation," it grants individuals the right to obtain an explanation of the decision reached and to challenge it. However, the scope of this right is limited to decisions made "solely" by automated processing and those that have legal or significant effects.
The interpretation and implementation of the "right to explanation" remain a subject of discussion among legal scholars and professionals. Some argue for a flexible and functional interpretation, enabling individuals to exercise their rights effectively. Others propose a narrow interpretation, suggesting that providing certain types of explanations may infringe on trade secrets and intellectual property rights.
To address these concerns, the concept of "counterfactual explanations" has been introduced. Counterfactual explanations provide individuals with limited information about the decision-making process, focusing on the factors that influenced the outcome without disclosing sensitive details. This approach aims to balance the need for transparency and the protection of proprietary information.
The ongoing dialogue and research in this area aim to establish a clear framework for the "right to explanation," ensuring that machine learning systems are accountable, transparent, and fair to individuals whose lives are significantly impacted by their decisions.
Stark Law: Applicability to Non-Medicare Patients Explained
You may want to see also
The role of machine learning in administrative law
Machine learning (ML) is increasingly being used in the legal industry, and administrative law is no exception. In the context of administrative law, machine learning can be categorised into two types: adjudication by algorithm and regulation by robot.
Adjudication by algorithm is useful when quantifiable data determines an outcome, such as eligibility for benefits. In this scenario, machine learning algorithms make inferences and predictions about data without being explicitly programmed. They "learn" from the data to produce an output through pattern recognition. This can help agencies make better and faster decisions by processing larger data sets than humans. For example, in the US, the Social Security Administration serves millions of people through online forms and plans to expand its platform.
Regulation by robot can be applied to improve traffic flow and reduce delays. An example is the City of Los Angeles, which uses an algorithm that synthesises large amounts of data to adjust traffic lights accordingly.
However, the adoption of machine-learning algorithms in administrative law is controversial. One of the main challenges is the potential for bias, especially when algorithms are trained on historical data that may reflect societal biases. Another challenge is transparency and interpretability, as complex models like deep neural networks often operate as "black boxes", making it difficult to understand and explain their decision-making processes.
To address these challenges, legal professionals and technologists must collaborate to develop strategies for mitigating bias and ensuring transparency and interpretability in ML models. Additionally, legal frameworks must adapt to establish clear accountability and responsibility for any errors or biases that may arise from using these technologies.
While machine learning in administrative law offers benefits such as increased efficiency and accuracy, addressing these challenges is crucial to ensure that the technology complements the cornerstones of fairness and efficiency in the legal system.
Congress and Slzndsr Laws: Who's Exempt?
You may want to see also
The use of machine learning in legal research
Machine learning is increasingly being used in the legal industry to automate and streamline processes, with applications in legal research, contract review, e-discovery, investigations, and more.
Applications of Machine Learning in Legal Research
Machine learning algorithms can be trained to identify trends, patterns, and correlations in legal data, enabling lawyers to make more informed decisions. This technology can be used to predict the likely outcomes of legal cases, assist in settlement negotiations, and provide insights that may not be immediately evident to human researchers.
For example, subscription software such as ROSS, Westlaw Edge, or Casetext use Natural Language Processing to recommend relevant case law or resources. These tools employ machine learning to help attorneys discover important information that would otherwise require hours of manual research.
Benefits of Machine Learning in Legal Research
- Increased Efficiency: Machine learning algorithms can analyze vast amounts of data much faster than human researchers, reducing the time and effort required for traditional legal research.
- Improved Accuracy: Machine learning can identify patterns and correlations in legal data, leading to more accurate predictions and informed decision-making.
- Enhanced Predictive Analysis: By identifying trends in historical data, machine learning enables lawyers to predict likely case outcomes and make more strategic choices.
- Automation: Machine learning can automate certain aspects of legal research, such as document review and data analysis, freeing up time for lawyers to focus on critical issues.
- Comprehensive Research: Machine learning algorithms can process and analyze large volumes of legal information, ensuring a more comprehensive and exhaustive research process.
Challenges and Ethical Considerations
While machine learning offers significant benefits in legal research, it also presents challenges and ethical considerations:
- Bias: Machine learning algorithms may inherit biases from the data they are trained on, potentially leading to discriminatory outcomes. Addressing and mitigating bias in machine learning models is crucial to ensuring fairness and transparency.
- Transparency and Interpretability: Complex machine learning models, such as deep neural networks, can be difficult to interpret, making it challenging for legal professionals to understand and explain their decisions.
- Accountability: Determining responsibility for errors or biases in machine learning models is complex, and legal frameworks must evolve to establish clear lines of accountability.
- Data Availability: The lack of large datasets for supervised learning in the legal domain poses a challenge for training machine learning models effectively.
In conclusion, machine learning has the potential to revolutionize legal research by providing efficient, accurate, and insightful analysis of legal data. However, addressing the challenges and ethical considerations is essential to ensure the responsible and effective use of this technology in the legal domain.
Applying for a Lawful Development Certificate: A Guide
You may want to see also
The application of machine learning in investigations and e-discovery
Machine learning has become an integral part of e-discovery, which involves identifying, collecting, and reviewing electronically stored information (ESI) to support legal proceedings. ESI can include data stored on devices such as laptops, smartphones, corporate email systems, social media platforms, and more. The vast amount of data involved in investigations makes it practically impossible to conduct a manual review. This is where machine learning comes into play.
At the start of an investigation, a machine learning algorithm can be set up to read documents, search for relevant keywords, and cluster them into groups based on their content. This process, known as "conceptual clustering" or "conceptual searching", helps investigators identify patterns and gain insights into the data. For example, if the algorithm is asked to identify information about tennis and football, it may also cluster documents containing details about other sports.
Machine learning can also assist in redacting personal information from documents, ensuring compliance with privacy laws and regulations such as GDPR. Additionally, machine learning can aid in the detection of anomalies or outliers in data, which is crucial for fraud detection and investigation.
One of the key advantages of using machine learning in e-discovery is the significant cost and time savings it offers. It can increase the speed of document review by 15-20% and reduce the total number of hours required for a review by up to 40%. However, it's important to note that the effectiveness of machine learning depends on the nature of the problem domain and the availability of data.
When using machine learning in investigations and e-discovery, it's essential to have a clear understanding of the methodology and how it works. This includes knowledge of the data being used, how it's collected, and any potential biases that may exist. Additionally, it's crucial to have the right technical skills and infrastructure to train and deploy machine learning models effectively.
In summary, machine learning plays a crucial role in investigations and e-discovery by providing efficient data processing, pattern recognition, and anomaly detection capabilities. However, it should be used in collaboration with human experts to ensure accurate and ethical outcomes.
The Legal System: Unfair to the Less Fortunate?
You may want to see also
The potential biases in machine learning
Machine learning (ML) models are not inherently objective. They are trained using datasets, and human involvement in the provision and curation of this data can make a model's predictions susceptible to bias.
There are several types of bias that can influence ML systems:
- Reporting bias: This occurs when the frequency of events, properties, and outcomes in a dataset does not accurately reflect their real-world frequency. For example, if a sentiment analysis model is trained on a dataset of book reviews that mostly reflect extreme opinions, it will struggle to predict the sentiment of more nuanced reviews.
- Historical bias: Historical data may reflect past societal inequalities and injustices. For instance, a housing dataset from the 1960s may contain home-price data that reflects discriminatory lending practices.
- Automation bias: This is the tendency to favour results generated by automated systems, even when they are less accurate than those produced by non-automated systems.
- Selection bias: This occurs when a dataset's examples are chosen in a way that does not reflect their real-world distribution. This can take the form of coverage bias, non-response bias, or sampling bias.
- Group attribution bias: This is the tendency to generalize characteristics of individuals to the entire group they belong to. This includes in-group bias, favouring members of one's own group, and out-group homogeneity bias, stereotyping members of groups one does not belong to.
- Implicit bias: This occurs when assumptions are made based on one's own experiences that do not apply more generally. For example, using a head shake as a feature to indicate "no" in a gesture recognition model, when in some regions, a head shake means "yes".
- Confirmation bias: This occurs when model builders process data in a way that affirms pre-existing beliefs and hypotheses.
- Experimenter's bias: This occurs when a model is trained until it produces a result that aligns with the builder's original hypothesis.
- Algorithm bias: This occurs when there is a problem with the algorithm that performs the calculations or processing for the ML computations.
- Sample bias: This occurs when the data used to train the ML model is not large or representative enough. For example, if a system is trained on data featuring only female teachers, it may conclude that all teachers are female.
- Prejudice bias: This occurs when the data used to train the system reflects existing prejudices, stereotypes, and faulty societal assumptions. For instance, using data about medical professionals that includes only female nurses and male doctors could perpetuate gender stereotypes.
- Measurement bias: This arises from underlying problems with the accuracy of the data and how it was measured or assessed. Training data that is not truly representative can skew the system's understanding.
- Exclusion or reporting bias: This occurs when an important data point is left out of the dataset. For example, incidents may go unreported or under-reported in police crime analytics due to victims failing to report them.
- Recall bias: This data quality bias occurs in the data labelling stage, where labels are inconsistently given through subjective observations.
To prevent biased models, organizations should use comprehensive and diverse data that is representative of different races, genders, backgrounds, and cultures. Data scientists should shape data samples to minimize bias, and decision-makers should evaluate when it is appropriate to apply ML technology. Models should be tested and validated to ensure they do not reflect biases, and additional tools can be used to examine and inspect them. It is also crucial to continually review and improve ML models as more feedback is received.
While the potential biases in ML are extensive, being aware of these risks and actively working to address them can help mitigate their impact.
Laws of Motion: Space Edition
You may want to see also
Frequently asked questions
Machine learning is a subset of artificial intelligence (AI). AI is a broader term for intelligent machines that can mimic human understanding. Machine learning is a specific element of AI that centres around data. It uses algorithms and statistics to find patterns within huge datasets.
Machine learning algorithms are divided into three categories: supervised learning, unsupervised learning, and reinforcement learning. Supervised learning involves labelled data that is classified to tell the machine the patterns to look for. Unsupervised learning involves unlabelled data, where the machine looks for any patterns and groups the data accordingly. Reinforcement learning involves the algorithm using a trial-and-error method to achieve a clear objective, receiving rewards or penalties for its attempts.
The law is still catching up with machine learning and artificial intelligence. However, regulations are being developed to ensure transparency and accountability. For example, the European Union has passed a regulation giving citizens the "right to an explanation" for decisions made by machine-learning systems. The legal industry is also exploring the use of machine learning to improve efficiency and reduce manual efforts, particularly in legal research, contract review, and investigations.